While operational opacity generated by machine learning algorithms presents a wide range of problems for ethics and computer science (Burrell 10), one type in particular may be unavoidable due to the nature of complex processes. The physical underpinnings of functional systems may be difficult to understand because of the way data is stored and transmitted. Just as patterns of neural activity seem conceptually distant from first-person accounts of subjective experiences, the missing explanation for why or how a DCNN arrives at a particular decision may actually be a feature of the system rather than a bug. Systems capable of storing or processing large amounts of data may only be capable of doing so because of the way nested relationships are embedded in the structure. Furthermore, many of the human behaviours or capacities researchers are trying to understand and copy are both complex and emergent, making them difficult to fully trace back to the physical level of implementation. When we do, it often looks strange and quite chaotic. For example, molecular genetics suggests various combinations of nucleotides give rise to different types of cells and proteins, each with highly specialized and synergistic functions. Additionally, complex phenotypes like disease dispositions are typically the result of many interacting genotypic factors in conjunction with the presence of certain environmental variables. If it turns out to be the case that a degree of opacity is a necessary component of convoluted functionality, we may need to rethink our expectations of how ethics can inform the future of AI development.
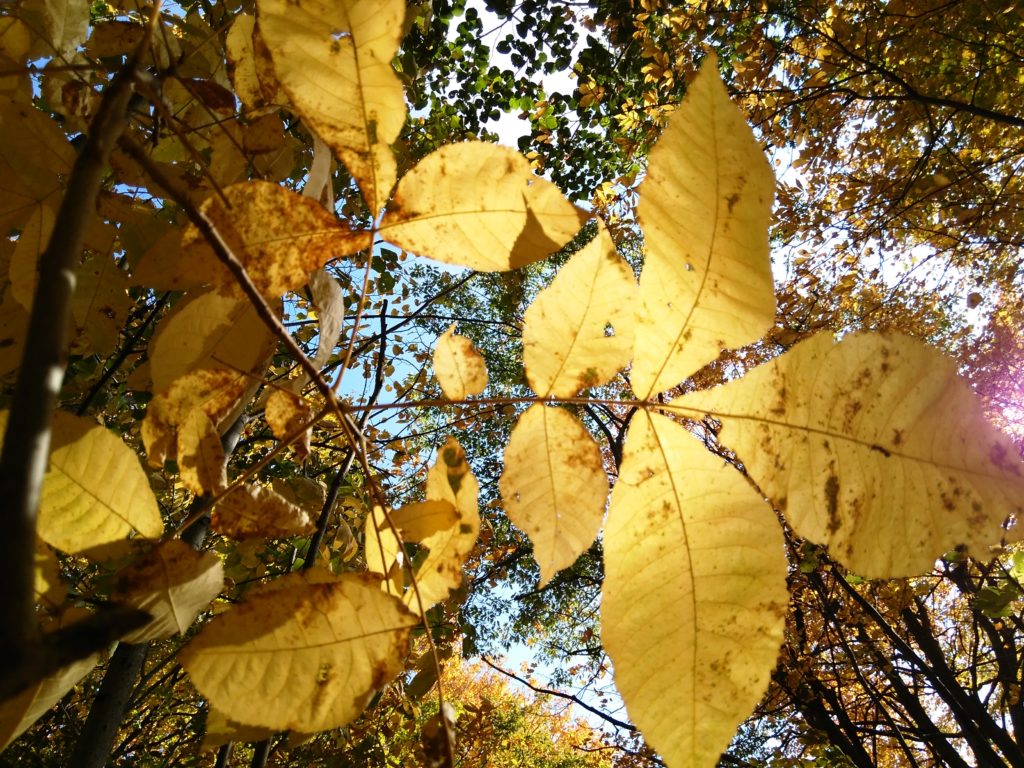
Works Cited
Burrell, Jenna. “How the machine ‘thinks’: Understanding opacity in machine learning algorithms.” Big Data & Society 3.1 (2016).