What does it mean to call something an example of “artificial intelligence” (AI)? There are a few different ways to approach this question, one of which includes examining the field to identify an overarching definition or set of themes. Another involves considering the meanings of the words ‘artificial’ and ‘intelligence’, and arguably, doing so enables the expansion of this domain to include new approaches to AI. Ultimately, however, even if these agents one day exhibit sophisticated or intelligent behaviours, they nonetheless continue to exist as artifacts, or objects of creation.
The term artificial intelligence was conceived by computer scientist John McCarthy in 1958, and the purported reason he chose the term was to distinguish it from other domains of study.1 In particular, the field of cybernetics which involves analog or non-digital forms of information processing, and automata theory as a branch of mathematics which studies self-propelling operations.2 Since then, the term ‘artificial intelligence’ has been met with criticism, with some questioning whether it is an appropriate term for the domain. Specifically, Arthur Samuel was not in favour of its connotations, according to computer scientist Pamela McCorduck in her publication on the history of AI.3 She quotes Samuel as stating “The word artificial makes you think there’s something kind of phony about this, or else it sounds like it’s all artificial and there’s nothing real about this work at all.”4
Given the physical distinctions between computers and brains, it is clear that Samuel’s concerns are reasonable, as the “intelligence” exhibited by a computer is simply a mathematical model of biological intelligence. Biological systems, according to Robert Rosen, are anticipatory and thus capable of predicting changes in the environment, enabling individuals to tailor their behaviours to meet the demands of foreseeable outcomes.5 Because biological organisms depend on specific conditions for furthering chances of survival, they evolved ways to detect these changes in the environment and respond accordingly. As species evolved over time, their abilities to detect, process, and respond to information expanded as well, giving rise to intelligence as the capacity to respond appropriately to demanding or unfamiliar situations.6 Though we can simulate intelligence in machines, the use of the word ‘intelligence’ is metaphorical rather than literal. Thus, behaviours exhibit by computers is not real or literal ‘intelligence’ because it arises from an artifact rather than from biological outcomes.
An artifact is defined by Merriam-Webster as an object showing human workmanship or modification, as distinguished from objects found in nature.7 Etymologically, the root of ‘artificial’ is the Latin term artificialis or an object of art, where artificium refers to a work of craft or skill and artifex denotes a craftsman or artist.8 In this context, ‘art’ implies a general sense of creation and applicable to a range of activities including performances as well as material objects. The property of significance is its dependence on human action or intervention: “artifacts are objects intentionally made to serve a given purpose.”9 This is in contrast to unmodified objects found in nature, a distinction first identified by Aristotle in Metaphysics, Nicomachean Ethics, and Physics.10 To be an artifact, the object must satisfy three conditions: it is produced by a mind, involves the modification of materials, and is produced for a purpose. To be an artifact, an object or entity must meet all three criteria.
The first condition states the object must have been created by a mind, and scientific evidence suggests both humans and animals create artifacts.11 For example, beaver dams are considered artifacts because they block rivers to calm the water which creates ideal conditions for a building a lodge.12 Moreover, evidence suggests several early hominid species carved handaxes which serve social purposes as well as practical ones.13 By chipping away at a stone, individuals shape an edge into a blade which can be used for many purposes, including hunting and food preparation.14 Additionally, researchers have suggested that these handaxes may also have played a role in sexual selection, where a symmetrically-shaped handaxe demonstrating careful workmanship indicates a degree of physical or mental fitness.15 Thus, artifacts are important for animals as well as people, indicating the sophisticated abilities involved in the creation of artifacts is not unique to humans.
Computers and robots are also artifacts given that they are highly manufactured, functionally complex, and created for a specific purpose. Any machine or artifact which exhibits complex behaviour may appear to act intelligently, however, the use of ‘intelligent’ is necessarily metaphorical given the distinction between artifacts and living beings. There may one day exists lifelike machines which behave like humans, however, any claims surrounding literal intelligence must demonstrate how and why that is; the burden of proof is theirs to produce. An argument for how a man-made object sufficiently models biological processes is required, and even then, remains a simulation of real systems.
If the growing consensus in cognitive science indicates individuals and their minds are products of interactions between bodily processes, environmental factors, and sociocultural influences, then we should to adjust our approach to AI in response. For robots intending to replicate human physiology, a good first step would be to exchange neural networks made from software for ones built from electrical circuits. The Haikonen Associative Neuron offers a solution to this suggestion,16 and when coupled with the Haikonen Cognitive Architecture, is capable of generating the required physiologicalprocesses for learning about the environment.17 Several videos uploaded to YouTube demonstrate a working prototype of a robot built on these principles, where XCR-1 is able to learn associations between stimuli in its environment, similarly to humans and animals.18 Not only is it a better model of animal physiology than robots relying on computer software, the robot is capable of performing a range of cognitive tasks, including inner speech,19 inner imagery,20 and recognizing itself in a mirror.21
So, it seems that some of Arthur Samuel’s fears have been realized, considering machines merely simulate behaviours and processes identifiable in humans and animals. Moreover, the use of ‘intelligence’ is metaphorical at best, as only biological organisms can display true intelligence. If an aspect of Samuel’s concerns related to securing funding within his niche field of study, and its potential to fall out of fashion, he has no reason to worry. Unfortunately, Samuel passed away in 199022 so he would not have had a chance to see the monstrosity that AI has since become.
Even if these new machines were to become capable of sophisticated behaviours, they will always exist as artifacts, objects of human creation and designed for a specific purpose. The etymological root of the word ‘artificial’ alone provides sufficient grounds for classifying these robots and AIs as objects, however, as they continue to improve, this might become difficult to remember at times. To avoid being deceived by these “phony” behaviours, it will become increasingly important to understand what these intelligent machines are capable of and what they are not.
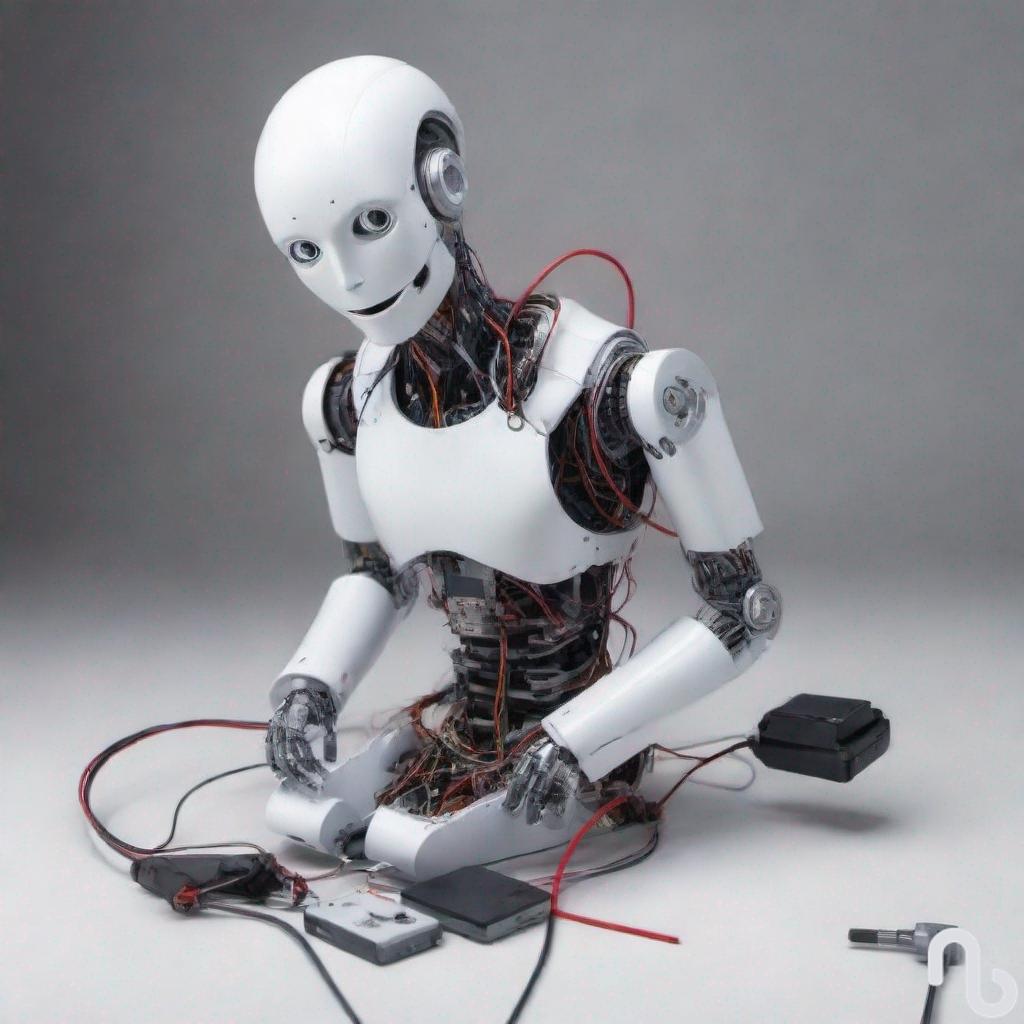
Works Cited
1 Nils J. Nilsson, The Quest for Artificial Intelligence (Cambridge: Cambridge University Press, 2013), 53, https://doi.org/10.1017/CBO9780511819346.
2 Nilsson, 53.
3 Nilsson, 53.
4 Pamela McCorduck, Machines Who Think: A Personal Inquiry Into the History and Prospects of Artificial Intelligence, [2nd ed.] (Natick, Massachusetts: AK Peters, 2004), 97; Nilsson, The Quest for Artificial Intelligence, 53.
5 Robert Rosen, Anticipatory Systems: Philosophical, Mathematical, and Methodological Foundations, 2nd ed., IFSR International Series on Systems Science and Engineering, 1 (New York: Springer, 2012), 7.
6 ‘Intelligence’, in Merriam-Webster.Com Dictionary (Merriam-Webster), accessed 5 March 2024, https://www.merriam-webster.com/dictionary/intelligence.
7 ‘Artifact’, in Merriam-Webster.Com Dictionary (Merriam-Webster), accessed 17 October 2023, https://www.merriam-webster.com/dictionary/artifact.
8 Douglas Harper, ‘Etymology of Artificial’, in Online Etymology Dictionary, accessed 14 October 2023, https://www.etymonline.com/word/artificial; ‘Artifact’.
9 Lynne Rudder Baker, ‘The Ontology of Artifacts’, Philosophical Explorations 7, no. 2 (1 June 2004): 99, https://doi.org/10.1080/13869790410001694462.
10 Beth Preston, ‘Artifact’, in The Stanford Encyclopedia of Philosophy, ed. Edward N. Zalta and Uri Nodelman, Winter 2022 (Metaphysics Research Lab, Stanford University, 2022), https://plato.stanford.edu/archives/win2022/entries/artifact/.
11 James L. Gould, ‘Animal Artifacts’, in Creations of the Mind: Theories of Artifacts and Their Representation, ed. Eric Margolis and Stephen Laurence (Oxford, UK: Oxford University Press, 2007), 249.
12 Gould, 262.
13 Steven Mithen, ‘Creations of Pre-Modern Human Minds: Stone Tool Manufacture and Use by Homo Habilis, Heidelbergensis, and Neanderthalensis’, in Creations of the Mind: Theories of Artifacts and Their Representation, ed. Eric Margolis and Stephen Laurence (Oxford, UK: Oxford University Press, 2007), 298.
14 Mithen, 299.
15 Mithen, 300–301.
16 Pentti O Haikonen, Robot Brains: Circuits and Systems for Conscious Machines (John Wiley & Sons, 2007), 19.
17 Pentti O Haikonen, Consciousness and Robot Sentience, 2nd ed., vol. 04, Series on Machine Consciousness (WORLD SCIENTIFIC, 2019), 167, https://doi.org/10.1142/11404.
18 ‘Pentti Haikonen’, YouTube, accessed 6 March 2024, https://www.youtube.com/@PenHaiko.
19 Haikonen, Consciousness and Robot Sentience, 182.
20 Haikonen, 179.
21 Robot Self-Consciousness. XCR-1 Passes the Mirror Test, 2020, https://www.youtube.com/watch?v= WE9QsQqsAdo.
22 John McCarthy and Edward A. Feigenbaum, ‘In Memoriam: Arthur Samuel: Pioneer in Machine Learning’, AI Magazine 11, no. 3 (15 September 1990): 10, https://doi.org/10.1609/aimag.v11i3.840.